The WorldStrat dataset.
Nearly 10,000 km² of free high-resolution and matched low-resolution satellite imagery of unique locations which ensure stratified representation of all types of land-use across the world: from agriculture to ice caps, from forests to multiple urbanization densities.
Each high-resolution image (1.5 m/pixel) comes with multiple temporally-matched low-resolution images from the freely accessible lower-resolution Sentinel-2 satellites (10 m/pixel).
The dataset is accompanied by:
🎓 A paper, published at NeurIPS 2022.
📃 A datasheet for datasets.
🔍 Several pre-trained super-resolution models.
🔧An open-source Python package to rebuild or extend the dataset, train and infer baseline algorithms, learn through abundant tutorials.
Preview high-resolution and 16 matching low-resolution:
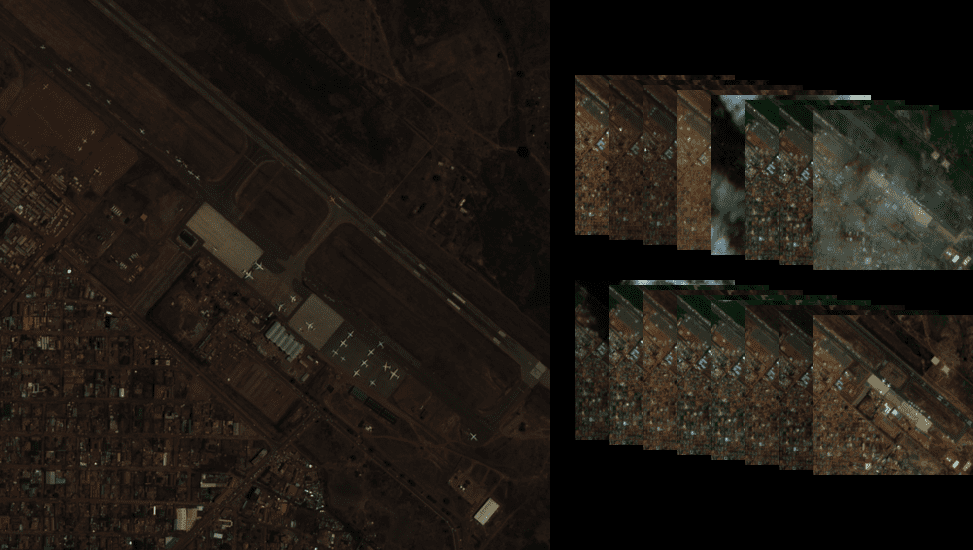
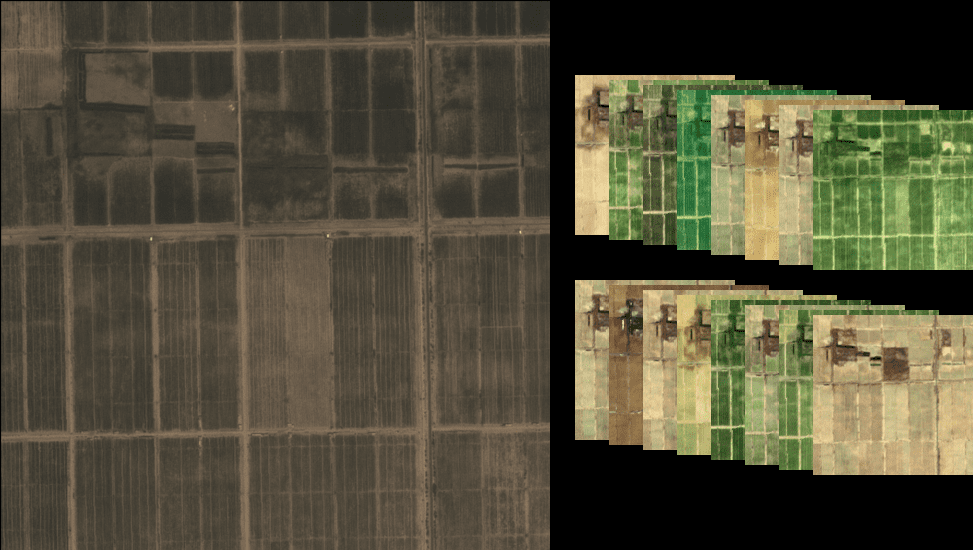
How to cite:
Plaintext:
J. Cornebise, I. Oršolic, F. Kalaitzis. (2022) High-Resolution Satellite Imagery: The WorldStrat Dataset – With Application to Super-Resolution, Advances in Neural Information Processing System 35, Proceedings of NeurIPS 2022.
BibTeX:
@inproceedings{
cornebise2022open,
title={Open High-Resolution Satellite Imagery: The WorldStrat Dataset {\textendash} With Application to Super-Resolution},
author={Julien Cornebise and Ivan Orsolic and Freddie Kalaitzis},
booktitle={Thirty-sixth Conference on Neural Information Processing Systems Datasets and Benchmarks Track},
year={2022},
url={https://openreview.net/forum?id=DEigo9L8xZA}
}